Explanation needed - definition of knowledge and knowldge models
Hi!
I am really new to the field and could use some explanations from fellow course participants with more theoretical experience in the field:
I took this line from the course syllabus because I have some questions:
1. How is knowledge defined in this course?
2. What are knowledge models and which one already exist?
3. What is knowledge modelling?
(I am only asking this because I don't want to google that and get zillions of websites related to knowldge, I just want an orientation, names or links)
Re: Explanation needed - definition of knowledge and knowldge models
Let me tackle the first one as I suspect each of these could lead into fairly complex discussion.
My definition of knowledge is informed by my view of learning as a social, distributed process (i.e. connectivism). Learning and knowledge are essentially the same thing - one a process the other a product.
Knowledge is a pattern of connectedness. (I tackled this in Knowing Knowledge in 2006: http://www.elearnspace.org/KnowingKnowledge_LowRes.pdf ...Stephen Downes has addressed connective knowledge in contrast to existing views of knowledge here: http://www.downes.ca/post/33034 ). Learning is the process of forming and pruning those connections. I've attached an image that, while a bit simplistic, gets at my view of knowledge.
I emphasize connectedness between concepts as a foundation of knowledge for two primary reasons:
First, in complex societies, facing complex problems, knowledge becomes increasingly specialized. In order to do anything - build a computer, find a virus causing a disease (i.e. SARS), design the 787 - we need to connect specialists. In putting together LAK11 (course and conference), the interdisciplinary/distributed attributes of knowledge were a key focus. I don't think the field will grow to its potential unless we have sociologists, psychologists, computer scientists, practitioners of learning and information sciences, etc. involved. In my EDUCAUSE presentation on Monday, I argued that the limited impact of early work with cognitive tutors can at least partly be explained by the lack of integration with other fields.
Secondly, my interests in learning/knowledge as connective drives my interest in analytics (the course subtitle is "analyzing what can be connected). Once we have a better understanding of how and why connections form, the influence of connection forming, the social factors that influence knowledge development, etc., we can begin to fine tune learning ecosystems, predict learner performance, and plan interventions. Connectivism necessitates learning and knowledge analytics.
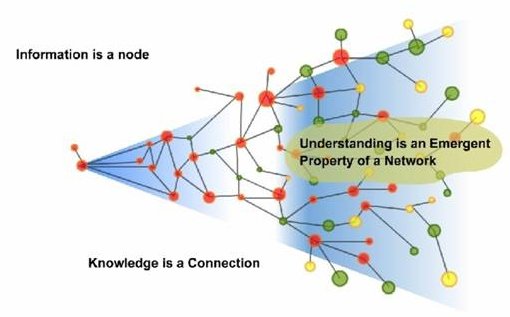
Re: Explanation needed - definition of knowledge and knowldge models
Re: Explanation needed - definition of knowledge and knowldge models
Beware these ideas about knowledge are unique to the course organizers and not shared in the industry or anywhere else. As far as I know, there never has been a refereed paper published. They are seen at best as highly speculative by most.
I am quite puzzled as how you could use these vague and incomplete ideas for knowledge analytics. Has there ever been any attempt by the MOOC organizers to measure how much knowledge has been acquired by participants in their course?
The truth is that I had never heard the term "knowledge analytics" before. They may have made it up. Not clear whether you can really ever claim to measure changes in knowledge levels.
It is not clear whether it makes any sense to introduce it. How can you pretend to measure knowledge in a human being? The models of knowledge that I know to have been directly evaluated are in artificial systems like computer expert systems.
Historically, people have preferred to stick to what they could measure with some validity/reliability. In the domain of instruction, Kirkpatrick, for instance introduced a four-levels of evaluation model (1994):
- Reaction - how the learners react to the learning process →learner-satisfaction
- Learning - the extent to which the learners gain knowledge and skills →learning-evaluation
- Behavior - capability to perform the learned skills while on the job →performance-evaluation
- Results - includes such items as monetary, efficiency, moral, etc. →impact-evaluation
Some links are provided in this (blog) article on Nuts and Bolts: How to Evaluate e-Learning
Knowledge is also abundantly discussed in the field of cognitive sciences. It is then typically associated with semantic content. Some discussion on different assumptions that can be made about the representation of that content can be found in this paper on the The Role of Cognitive Architecture in Theories of Cognition. This is not the classical view. Instead, a paper that considers the notion of distributed representations of the type discussed by Downes.
Re: Explanation needed - definition of knowledge and knowldge models
http://www.nwlink.com/~donclark/hrd/isd/kirkpatrick.html
Perhaps most relevant, Level Two - Learning
"This is the extent to which participants change attitudes, improve knowledge, and increase skill as a result of participating in the learning process. It addresses the question: Did the participants learn anything? The learning evaluation require some type of post-testing to ascertain what skills were learned during the training. In addition, the post-testing is only valid when combined with pre-testing, so that you can differentiate between what they already knew prior to training and what they actually learned during the training program."
Re: Explanation needed - definition of knowledge and knowldge models
For instance: http://lmgtfy.com/?q=knowledge+representation+lecture+filetype%3Apdf
Re: Explanation needed - definition of knowledge and knowldge models
I cannot see any value in the connectivism from any of these perspectives.
It has no value to me as a cognitive psychologist. A Learning Theory is a Theory of how skills and knowledge are acquired. And as such, it must refer to mental constructs. As such, theories of learning are proposed and evaluated by cognitive psychologists or cognitive scientists, who have for primary job to try and understand how knowledge is represented and processed in the mind.
Connectivism as it is currently formulated presents no value to the field of cognitive sciences. It is not presented in a form that would allow scientific debate of any nature. It mostly proposes some wild speculations based on some rapidly baked (mis)representations of the ideas in the literature. In the case of Downes writings, it amounts to what I, personally, as an academic with knowledge in the topics discussed, see as intellectual dishonesty (misrepresentations amounting to false claims).
It has no value to me as an elearning practitioner. It has strictly nothing to say about strategies for instructional design. Vague and very abstract ideas are introduced that cannot really be turned into implementations.
If you take this paper "What connectivism is", by Downes, http://halfanhour.blogspot.com/2007/02/what-connectivism-is.html, you will rapidly find out that it is phrased to deliberately avoid any accountability. Because it makes no specific hypothesis of what you get from being connected, there is no way to run any form of evaluation. The responsibility is fully on the learner for any "growth" (undefined) to happen.
You could retort. Not true, an implementation is possible. These MOOC are an example. But are MOOC an implementation of instruction? Do you actually have evidence that learning does happen in MOOC? More importantly, do you have any evidence that MOOC provide a more efficient way of delivering instruction than existing methods (videos available anytime like TED, academicearth.org, or open courseware initiative). I personally find MOOC to be a huge time investment for very little if any return. Are MOOC any better at promoting long-term connectedness than other media. Can you establish, with enough certainty, that MOOC leads to more than temporary noise going through the connections, without any long-term transformation of any type?
In the promo material, it is said that there is no assignment. No evaluation of what learners take from MOOC courses. There is no stated learning objective, no stated learning outcome that you can use to evaluate whether you are or not successful.
I has no value to me as a software developer or an information architect. Though connectivism sees connections as critical, they seem to loose track of the fact that connections truly exist only if people keep in contact. Any benefit of being connected stops when people stop to connect. MOOC has huge drop outs rates. Yet, there is no discussion on the strategies that can be followed to keep people engaged. Connectivism doesn't find useful to cover the issue of engagement, despite the fact that has been amply covered in other fields like social gaming. Excellent video : http://www.wetware.co.nz/blog/2010/08/jesse-schell-future-of-games-dice-2010-design-outside-the-box-presentation/
My development community has nothing to learn from connectivism. It already organized itself socially, ensuring rapid and efficient transfer of quality knowledge between practitioners. Stack overflow is a good example of it being well done: http://stackoverflow.com/.
I honestly am baffled as to what connectivism's apparent attractiveness could have to say about the very poor investment in the notion of community of practice in academia.
The expert model and practices like publication of papers that provides very symbolic, not easily decomposable units of knowledge, could lead academia to start to evolve less rapidly than other fields that already have embraced more egalitarian social structures.
And the irony of all, is that the persons who claim that emergent knowledge should be encouraged, simply go on with good old practices.
Teacher-led video courses, 10 pages long blog posts, 100 pages long books. They choose to share their knowledge in a way that cannot be decomposed, remixed, reused.
Instead of MOOCs, with experts on the stage, you should set up a website like stackoverflow, an actual community of practice
- http://stackoverflow.com/
- http://programmers.stackexchange.com/
- http://webmasters.stackexchange.com/
- http://uxexchange.com/questions/
- http://math.stackexchange.com/
- http://cooking.stackexchange.com/
These websites have value. Social connectedness has great value. Informal learning has value. Tangential learning has value.
Connectivism may have temporary value in the sense that they get more academics / education practitioners wonder about the role of social network in knowledge construction. But it has no long-term value to the community. it will soon be made completely irrelevant once websites like stackexchange start to appear and be used by the community.
To be fair, that there is a delay is expected. Developers typically have limited social connectivity in their direct environment (small teams, sometimes freelancing). Academics or eduction practitioners typically already have a huge social network and a very established community of practice. They have less reasons to try and create one online.
Hopefully, though, this will happen.
Re: Explanation needed - definition of knowledge and knowldge models
Some very recent papers on issues relevant to the course, published in academic journals (cognitive sciences at large), all published in "Trends in Cognitive Sciences", a journal that deliberately attempt to provide rather short (5 pages maximum) and accessible reviews (i.e., accessible to students or researchers in another field of expertise) of the latest advances in the field.
But before, you need to be aware of an important notion, the one of level of analysis.
Reproduced from Griffiths and al.
Most approaches to modeling human cognition agree that the mind can be studied on multiple levels. David Marr [1] defined three such levels:
- a ‘computational’ level charac- terizing the problem faced by the mind and how it can be solved in functional terms;
- an ‘algorithmic’ level describing the processes that the mind executes to produce this solution;
- and a ‘hardware’ level specifying how those pro- cesses are instantiated in the brain.
Cognitive scientists disagree over whether explanations at all levels are useful, and on the order in which levels should be explored. Many connectionists advocate a bottom-up or ‘mechanism-first’ strategy (see Glossary), starting by exploring the problems that neural processes can solve. This often goes with a philosophy of ‘emergentism’ or ‘eliminativism’: higher- level explanations do not have independent validity but are at best approximations to the mechanistic truth; they describe emergent phenomena produced by lower-level mechanisms. By contrast, probabilistic models of cognition pursue a top-down or ‘function-first’ strategy, beginning with abstract principles that allow agents to solve problems posed by the world – the functions that minds perform – and then attempting to reduce these principles to psychological and neural processes. Understanding the lower levels does not eliminate the need for higher-level models, because the lower levels implement the functions specified at higher levels.
Cognitive culture: theoretical and empirical insights into social learning strategies
http://lalandlab.st-andrews.ac.uk/pdf/Publication158.pdf
Abstract: Research into social learning (learning from others) has expanded significantly in recent years, not least because of productive interactions between theoretical and em- pirical approaches. This has been coupled with a new emphasis on learning strategies, which places social learning within a cognitive decision-making framework. Understanding when, how and why individuals learn from others is a significant challenge, but one that is critical to numerous fields in multiple academic disci- plines, including the study of social cognition.
Social transmission of learning is further discussed by these authors in: http://lalandlab.st-andrews.ac.uk/pdf/Publication157.pdf
Letting structure emerge: connectionist and dynamical systems
approaches to cognition
http://psychology.stanford.edu/~jlm/papers/McCEtAl10TiCS_LettingStructureEmerge.pdf
For a summary of the latest views on connectionist models of knowledge represetnation
Also from this author: Emergence in Cognitive Science, http://psychology.stanford.edu/~jlm/papers/McClellandIPTOPiCSEmergence.pdf
A precis on semantic cognition (or the representation of meaning in the mind), connectionist perspective: http://psychology.stanford.edu/~jlm/papers/RogersMcC08BBSFinalProof.pdf
An excellent discussion on the role of models in cognitive sciences http://psychology.stanford.edu/~jlm/papers/McClelland09PlaceOfModelingtopiCS.pdf
Probabilistic models
Probabilistic models of cognition: exploring representations and
inductive biases
http://www.psychology.adelaide.edu.au/personalpages/staff/amyperfors/papers/griffithsetal10tics-probabilisticmodelsofcognition.pdf
Abstract: Cognitive science aims to reverse-engineer the mind, and many of the engineering challenges the mind faces involve induction. The probabilistic approach to modeling cognition begins by identifying ideal solutions to these inductive problems. Mental processes are then modeled using algorithms for approximating these solutions, and neural processes are viewed as mechanisms for imple- menting these algorithms, with the result being a top- down analysis of cognition starting with the function of cognitive processes. Typical connectionist models, by contrast, follow a bottom-up approach, beginning with a characterization of neural mechanisms and exploring what macro-level functional phenomena might emerge. We argue that the top-down approach yields greater flexibility for exploring the representations and inductive biases that underlie human cognition.
Large-scale brain networks in cognition: emerging methods and principles
http://ftp.ida.liu.se/~729G01/mtrl/Bressler.pdf
Abstract: An understanding of how the human brain produces cognition ultimately depends on knowledge of large-scale brain organization. Although it has long been assumed that cognitive functions are attributable to the isolated operations of single brain areas, we demonstrate that the weight of evidence has now shifted in support of the view that cognition results from the dynamic interactions of distributed brain areas operating in large-scale networks. We review current research on structural and functional brain organization, and argue that the emerging science of large-scale brain networks provides a coherent framework for understanding of cognition. Critically, this framework allows a principled exploration of how cognitive functions emerge from, and are constrained by, core structural and functional networks of the brain.
General cognitive principles for learning structure in time and space
http://ibis.tau.ac.il/twiki/pub/Zoology/Lotem/PublicationsList/TICS_2010_ACCESS_online_version.pdf
Abstract: How are hierarchically structured sequences of objects, events or actions learned from experience and represented in the brain? When several streams of regularities present themselves, which will be learned and which ignored? Can statistical regularities take effect on their own, or are additional factors such as behavioral outcomes expected to influence statistical learning? Answers to these questions are starting to emerge through a convergence of findings from naturalistic observations, behavioral experiments, neurobiological studies, and computational analyses and simulations. We propose that a small set of principles are at work in every situation that involves learning of structure from patterns of experience and outline a general framework that accounts for such learning.
They describe their ACCESS model to structure: Align Candidates, Compare, Evaluate Statistical/Social Significance: temporally constrained, socially embedded learning
Herding in humans
(no freely accessible PDF - http://dx.doi.org/10.1016/j.tics.2009.08.002)
Abstract: erding is a form of convergent social behaviour that can be broadly defined as the alignment of the thoughts or behaviours of individuals in a group (herd) through local interaction and without centralized coordination. We suggest that herding has a broad application, from intellectual fashion to mob violence; and that understanding herding is particularly pertinent in an increasingly interconnected world. An integrated approach to herding is proposed, describing two key issues: mechanisms of transmission of thoughts or behaviour between agents, and patterns of connections between agents. We show how bringing together the diverse, often disconnected, theoretical and methodological approaches illuminates the applicability of herding to many domains of cognition and suggest that cognitive neuroscience offers a novel approach to its study.
There is more info on C. Frith's page
"In the last 20 years we have learned much about the social brain. This knowledge has derived from brain imaging and the study of psychiatric and neurological patients. Many of the pioneers of these studies have presented their latest results at this meeting and two major processing systems stand out in terms of the amount of interest they received. One of these is the brain system that allows us to mentalise (have a ‘Theory of mind’); the other is the brain's mirror system or rather, systems. Until recently we thought that mentalising was a high level executive skill that required mental effort. However, new data shows that there is also an implicit and largely automatic component of mentalising and therefore, exploring the relationship between implicit and explicit mentalising is an important topic for the future. The relationship between the mentalising system and the brain’s mirror systems remains a controversial topic. In order to resolve this controversy we need to develop a computational account of the mentalising process. One idea is that the mirror system can be used to make predictions about an observed person’s next movement. This generates a prediction error, which the mentalising system can use to update representations of intentions and other mental states. However, I suggest that we need to explore the idea that there is a fundamental conflict between mirror and mentalising systems. Through a form of contagion, mirror systems optimise interactions when the people involved have common knowledge, goals and intentions. In contrast, the mentalising system monitors the differences between the knowledge and intentions of the self and the knowledge and intentions of others. For optimum functioning of this process, social contagion must be suppressed."
Mirror neuron system refers to research on primates that suggest the evidence of mirror neurons, neurons that are active both when a monkey observes an action and when it executes the same action. (http://en.wikipedia.org/wiki/Mirror_neuron)
Early word-learning entails reference, not merely associations
http://groups.psych.northwestern.edu/waxman/documents/WaxmanGelmanTICS2009.pdf
Conclusion: "We have underscored that two metaphors – child-as-data- analyst and child-as-theorist – are at play in word-learn- ing and conceptual development. As infants and young children build a repertoire of concepts and acquire words to describe them, they take advantage of both perceptual and conceptual information, and rely upon both the rudi- mentary theories that they hold and the statistics that they witness. Our goal in writing this article is to empha- size that our theories of acquisition should do the same (Box 3)."
Social psychology as a natural kind
http://www.wjh.harvard.edu/~scanlab/papers/2009_socialpsychology_TiCS.pdf
Conclusion: "By increasingly adopting the methods of cognitive neuro- science, social psychologists have discovered a previously unsuspected correspondence among many of the important phenomena at the core of the field. Such observations underscore the unique power of functional localization methods, such as neuroimaging, to uncover links among researchers who once believed themselves to be studying disparate empirical issues, but who we now understand to have been probing different manifestations of a common underlying system. This neurally inspired ‘lumping’ of seemingly disparate phenomena promises not only to help underscore what makes social psychology distinctive but also suggests the need to rethink the assumption that the field studies phenomena at a ‘higher’ or more ‘macro’ level than cognitive psychology. Rather than equating the study of social phenomena with a particular level of analysis, these findings suggest a view of social psychology as a unique branch of cognitive science, specialized for examin- ing a distinct and natural kind of approximate, shifting and internally generated – in other words, ‘fuzzy’ – cognitive operations"
Re: Explanation needed - definition of knowledge and knowldge models
thank you for your list of references, and your elaborate critique on Connectivism. I have used the term very often to describe a way of learning that involves the creation of a network not only of neurons, but of things outside of the body. In the end, this happens through acquiring skills in the brain that enables you to do this better than others, so in that respect you are right. On the other hand, it can be useful to draw the attention to this kind of learning (use of technology and network), rather than internalizing the information.
PS. I find the StackExchange sites very good examples, I use them all the time as well.
Re: Explanation needed - definition of knowledge and knowldge models
Learning through social interaction. Yes, it is important to draw the attention to this. But all you need for that is to refer to the notion of social learning.
Connectivism comes with unwarranted claims that are not supported by knowledge or evidence or knowledge in related fields (instructional design, cognitive psychology, neuroscience, network modelling).
"the creation of a network not only of neurons, but of things outside of the body"
Where connectivism gets it wrong is that it is not the creation of the network or the addition of connections that matters. What matters is how that network gets configured.
A critical stage in development, between childhood and adolescence is synaptic pruning. The brain reorganizes and eliminates many synaptic connections. "This pruning makes the brain's information processing more efficient and powerful while consuming less energy" http://www.sciencedaily.com/releases/2006/12/061207160458.htm
The brain breaks down its weakest connections preserving only those that experience has shown to be useful. The statement "use it or lose it" is apt here. The synapses that carry the most messages get stronger and the weaker, less used ones get cut out.
It goes the same way in connectionist networks that the connectivist theory was directly inspired from. The process of learning is not via the creation of a network as large as possible possible. Networks that grows connections at infinitum have been shown to be unable to learn. This notion shows up as well in a very different domain, human networks, with Dunbar's number.
"... there is a cognitive limit to the number of individuals with whom any one person can maintain stable relationships, that this limit is a direct function of relative neocortex size, and that this in turn limits group size ... the limit imposed by neocortical processing capacity is simply on the number of individuals with whom a stable inter-personal relationship can be maintained."
It is important to add information about the context in which this has been written. You can find more info here: http://www.lifewithalacrity.com/2004/03/the_dunbar_numb.html and you have also to bear in mind that Dunbar numbers is about strong ties, strong connections.
That doesn't mean that weak ties cannot add any extra value whatsoever http://socialmediatoday.com/SMC/169132. But overall, what defines how well you do is not the existence of connections. It is about how connections get to be configured as a consequence of your learning.
Connectionist Networks that only have connections strengths that are weak or medium perform excessively poorly. At the start of a neural network modeling experiment, all the connections are random, about 0.5 (with minimum values of 0 and 1) and the performance is absolute crap. Performance improves as some strong ties get to emerge. Put another way, is about how your learning process gets to configure these connections. And connectivism has strictly nothing to say about that. It assumes it's about the connections, not about the learning.
Connectivism makes the assumption that learning is in the connections because they tend to use the word learning as it was a synonym for knowledge / information.
It isn't.
What is critical to most theories in cognitive psychology is the distinction between the notion of knowledge an the one of process. Things outside your body is knowledge, representations, information that you can access to on demand. Learning is as a process.
Take a connectionist or neural network. A network of units heavily interconnected. What is encoded in the connections is the knowledge, in the form of weight of the connection that link two units. But the reason any learning happens at all is because each unit is made of a mini-processor. A very simplified one. A pattern is presented to the first layer of the network. The information propagate through the different layers, function of the weight of the connections between units, it reaches the final (output) layer. The pattern of activation over the output layer, as produced by the network is compared to the expected one (what should have been a correct answer). The comparison takes place at the level of each unit. The unit compares its level of activity to the expected one and modify the weight of any connection reaching this unit as a function of the size of the error signal. The function is non linear, connection weights between 0.3 and 0.7 are quite similar to one another. Key knowledge is captured by connection weights that go towards 0 (this connection has no value for predicting the correct response) or 1 (this connection is particularly useful for improving performance).
Knowledge is distributed over the connection. But the network gets to improve its performance over time (learn) thanks to a learning process that exists at the level of each unit (each unit is in fact a micro-processor that does some very basic computation).
Knowledge is outside your body. Connections give you access to that knowledge. But the process of learning is at the level of yourself as a unit.
Of course, you can change the level of analysis and then treat a group of person as a unit in a bigger network. The learning done by group A vs the one done by group B. But then, you are now discussing the learning in each group, not the learning in each individual. Learning can be described at very different levels of analysis. You can even have machine learning, learning that happens in non human entities. But you have to be clear, from the start, as to what is the level of analysis that you are considering. Connectivism permanently jumps from one level to the other without any warning.
Try to replace learning with another cognitive activity like speaking or listening. This would give "whenever you speak or write, this involves the creation of a network not only of neurons, but of things outside the body." The output, sound waves, written transcripts gets propagated outside of your body. But the processes responsible for the creation of these waves or transcripts are very much inside your "mind". Remove the source, your mind and what powers it, the brain, and no speaking can be produced.
A robot or an artificial system can speak. That both the robot and yourself are within a shared environment and both of you can realize the same performance doesn't mean that the environment explains your ability to speak. Speaking remains possible if you substitute one environment with a completely different one.
The same way, if I cut you out of your social network and move you to another environment, what you have learned so far doesn't disappear. A good example is moving from one country to another in a pre-internet era. The social group is very different. New experiences get created. But the learning that had been done through the connections you had in the first country doesn't go away. Over time, the network will get reconfigured. Visiting your home country 10 years later, you will have forgotten some names, some faces and some places. Make it another 10 years, and In my case, you will have started to loose your fluency in your native language. But you most of it would have remained in your memory, despite the absence of contact with the social network in which that knowledge was acquired.
Sure, there are plenty of processes available to you to access the knowledge of you social network, web 2.0 tools in particular. Twitter shout outs can be particularly effective. These processes without doubt assist your learning. In some case, it can be claimed that they augment it. But they are not "learning" itself. Learning was defined as the process responsible for the permanent storage of information in your brain. A term that better describes the complete experience (access to network + storage of the newly acquired information in your mind) is knowledge acquisition.
I am not saying that it would not be useful to introduce a term with a broader meaning. I am only saying that learning is a term that has an accepted meaning. Learning refers to the lasting changes in *a person's* behavior. Learning was defined in reference to a person. Connectivism at best is a theory of knowledge access, not a theory of learning.
Once a person has an impairment that prevents them to learn new things (dementia, short term memory loss as shown in the movie Memento), they can have as many connections as they want. The won't be able to learn, that is form representations in their mind. They have lost the process that will let them form new permanent representations. The official term for this is anterograde amnesia.
What the guy in Memento does is store the knowledge outside of his mind, on his body, via tattoos. He can't do learning anymore. What he can do is use other mental processes like reasoning to analyse the information written all over his body. Bear in mind, that this is a work of fiction; an interesting real case is patient HM - http://en.wikipedia.org/wiki/HM_(patient)
To be fair, somehow, it is a bit circular to say that learning is what happens in the mind when the notion of mind was introduced as an abstract entity where learning and other mental processes take place. It's where cognition happens. The "totality of conscious and unconscious mental processes and activities". That abstraction was mostly introduced to allow analysis at a level higher than the one of the neurons, allowing discussions in terms of functionalities and higher level representations (sensation, perception, attention, memory language; lexical and semantic knowledge). The difference between cognitivism and connectivism is the choice of the former to follow a principled and scientific approach. In cognitive psychology and other fields, theories are characterized by precise assumptions about the representations and processes at play and supported by working models and a variety of evidence, coming from a variety of fields, neuroscience, experimentation on normal participants, impairments.
Connectivism is not constrained by any scientific rigour. If you remove the notion of social learning, which was introduced long before and discussed in much more depth by others, all there is to connectivism is some very wild imaginings not supported by evidence.
A better term is collective intelligence. It comes from a book "Collective Intelligence: Mankind's Emerging World in Cyberspace", by Pierre Lévy and published in 1994. I haven't re-read it recently (I should). It may have dated. He predicted the social web phenomenon at a time where the internet had barely started to be used in universities (before making it to the general public). To quote Levy, "It is a form of universally distributed intelligence, constantly enhanced, coordinated in real time, and resulting in the effective mobilization of skills...No one knows everything, everyone knows something...". Intelligence for Levy is a combination of skills, understanding and knowledge. Skills are what we develop when we interact with physical things; our relations with signs and information give us knowledge; our interaction with others gives us understanding." http://www.amazon.com/Collective-Intelligence-Pierre-Levy/dp/0306456354
These ideas had been around for some time. The credit you can give them is to have used the reach of the web and web 2.0 tools to hand out a word to persons who were trying to put one on their experience.
These ideas are worth spreading. But you have to beware about using a term that is associated with some wild imaginings. The use of term of connectivism is likely to cause a backlash from the instructional design and academic communities.
It's safer to use the terms of collective intelligence and social learning (which really is a shortcut for learning-through-social interaction the same way mobile learning is a shortcut for learning-on-mobile devices).